This is the second half of a two-part Lab Long Read on a recent collaborative project between Policy Lab and the Department for Transport (DfT) on the policy consultation process. The first part described the motivation for the project and the kinaesthetic mapping approach we used to derive novel insights about policy consultations. This second part describes our conclusions regarding the role that data science can play in the policy consultation process.
Our analysis of the consultation map, pain points, and subsequent prototyping/user testing, identified the potential for data science tools in four ways: real-time demographic analysis of responses; issue mapping; sentiment analysis; and question-setting. Each of those is described in turn.
Real-time demographic analysis of consultation responses
The ‘Participation Live Flow’ prototype (see image below) shows how data science tools could provide policy makers with a real-time demographic analysis of responses to consultations, including by location, gender, ethnicity and age. This would depend on respondents providing demographic information (and consent for its use), and appropriate data protection laws would apply.
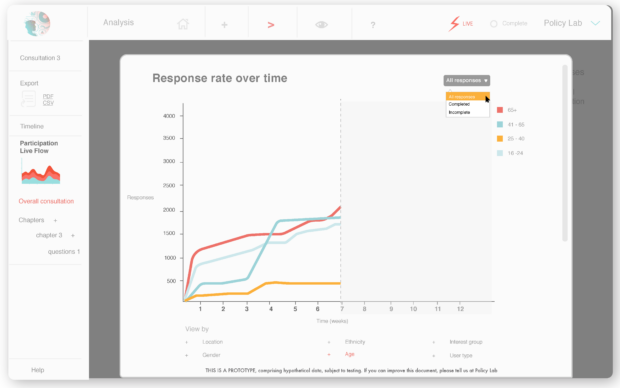
The live demographic data could have a number of policy applications. For example, policy makers could identify in real-time if certain population groups were underrepresented in the consultation, and then target further communication and engagement appropriately. When analysing the consultation results, policy makers could consider whether certain demographic groups were more likely to answer consultation questions in different ways and thus be disproportionately affected by policy proposals. This could enable policy makers to address policy biases or impacts on inequality.
Top issues arising from the consultation
The ‘Top Themes’ prototype tool allows policy makers to quickly and impartially identify key issues emerging from a consultation. It identifies consultation responses which have most in common with each other and allocates them into ‘theme groups’, which are then ranked according to their prevalence. Clicking on a theme group (second image below) allows the policy maker to quickly investigate a group of similar responses and provide an impartial analysis of the key issues emerging, either from the overall consultation or on a question-by-question basis.
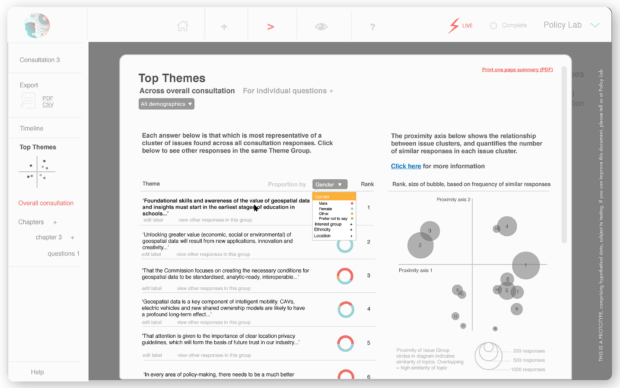
The Top Themes prototype tool aims to address a frequently cited pain point: the reading and synthesis of thousands of consultation responses (and often tens of thousands of individual question responses) into key categories or themes. This synthesis is important because it turns vast quantities of raw information into a set of issues that policy makers can address. It also helps identify what the majority of responses say and which are outliers. However, it is a hugely resource-intensive process, and is, by its very nature, subjective: the first responses may have a disproportionate impact on how the rest are sorted, whilst team members may have different interpretations of how responses should be categorised.
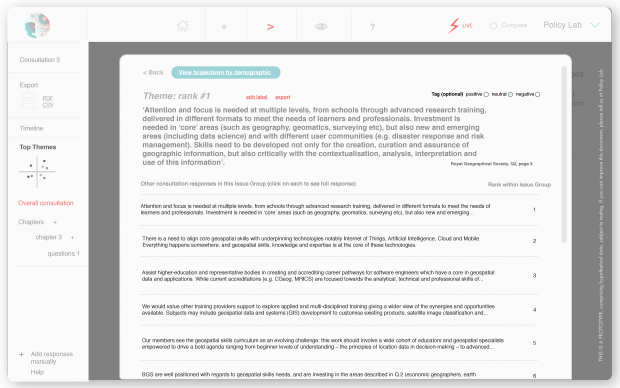
The aim of the Top Themes prototype tool is designed to reduce the heavy workload of categorisation and sorting stage, and free policy makers to focus on high value work, such as creatively developing policy ideas and considering the legal, technical and political implications of the main themes of response.
Using data science to analyse emotions in consultation responses
Another way that data science can support policy making is via ‘sentiment analysis’. Whilst the Top Themes prototype reduces subjectivity in analysis, the sentiment analysis prototype embraces the emotional quality of responses. Similar to Top Themes, the data science tool works through natural language processing, but considers emotional language in responses. It then sorts these according to Plutchik’s wheel of emotions which include anger, anticipation, disgust, fear, joy, sadness, surprise and trust (see image below).
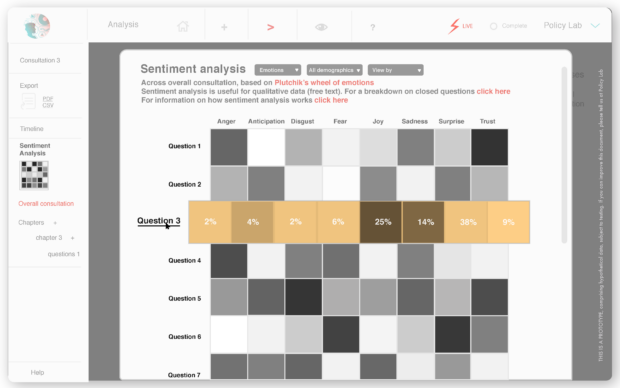
There are a number of ways in which better understanding emotion can improve policy making. Knowing if people feel scared, angry, sad or surprised about policy proposals can provide valuable intelligence to help refine the delivery of policies and develop more effective communication strategies. Understanding where public reaction veers into anger is particularly important, as it may require different responses compared to other negative emotional reactions. From a workforce health and wellbeing perspective, sentiment analysis could play an important role in helping managers support their staff when working on particularly controversial or sensitive topics.
More flexible and open responses to consultations
So far, the benefits have focused on the analysis side of the consultation, perhaps inevitable given the project brief to consider data science. There will be indirect benefits to citizens (for example through more efficient use of public funds and improved policy making), but are there direct benefits to citizens, especially those responding to consultations?
Data science has the potential to empower citizens to respond to consultations in more open and flexible ways. Consultations are currently fully digitalised, and most respondents respond via online portals. This allows quick digitalisation of results if questions are in the form of yes/no or multiple choice. Not all consultation questions can or should be asked in such a way. Policy makers are faced with a trade-off between framing questions as multiple choice or the heavy administrative workload if questions are posed as ‘open’ - which may be more appropriate in certain cases. Data science tools overcome this dilemma through their use of natural language processing which can read large volumes of free text, and extract meaning and insight from that text. Closed questions may still play an important role in consultations, but this functionality could open up creative ways of framing consultation questions.
In summary, quality assured data science could bring a consistency of approach to the analysis of consultations, free up policy makers and analysts to do those jobs that humans are best at carrying out (such as interpreting complex or nuanced responses), provide budget savings for government departments and bring innovative approaches into the analysis.
So what next?
Policy Lab has prototyped and tested these tool to provide user insight to data scientists who may want to develop them further. The data science team in DfT are now incorporating these findings into their work. You can contact them via the Policy Lab email address to find out more. But if you are a budding data scientist who wants to get involved with a key part of the policy development process, perhaps you would like to incorporate some of these user insights into your next tool?
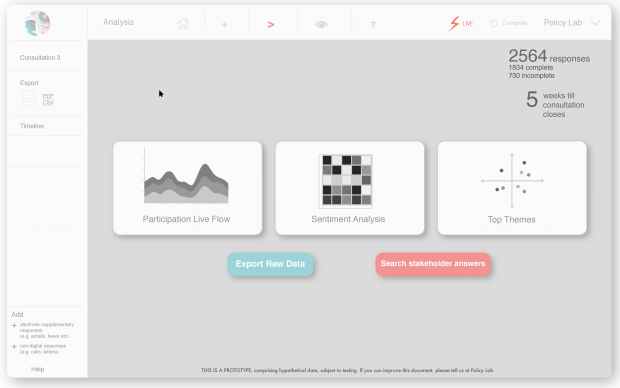
Leave a comment