This post sets out Collective Intelligence Lab’s reflections on our experiments to date and how this approach could be applied to complex policy areas such as climate change.
What is collective intelligence?
At Policy Lab, we strive to radically improve policymaking by experimenting with innovative, people-centred methods. These approaches are well suited to complex, persistent and systemic challenges like those faced by policymakers working on aspects of climate change - as we set out in recent posts about art in policy, systems mapping and co-design.
In November 2021 we established a Collective Intelligence Lab (CILab), with the aim of improving policy outcomes by tapping into collective intelligence (CI). We define CI as the diversity of thought and experience that is distributed across groups of people, from public servants and domain experts to members of the public. We have been experimenting with a digital tool, Pol.is, to capture diverse perspectives and new ideas on key government priority areas. To date we have run eight debates on issues as diverse as Civil Service modernisation, fisheries management and national security. Across these debates over 2400 civil servants, subject matter experts and members of the public have participated. We will be blogging about some of these areas in coming months. This post sets out our approach to CI so far, and describes three ways in which we think it helps to develop policy in complex settings.
Our approach to collective intelligence at CI Lab
Pol.is is an open source online platform that invites participants to enrich a debate by adding their viewpoints to a small number of initial seed statements, by submitting their own statements and voting on each others’ perspectives (see image below). Participants can therefore frame the discussion more in their own terms than they can in many other modes of engagement.
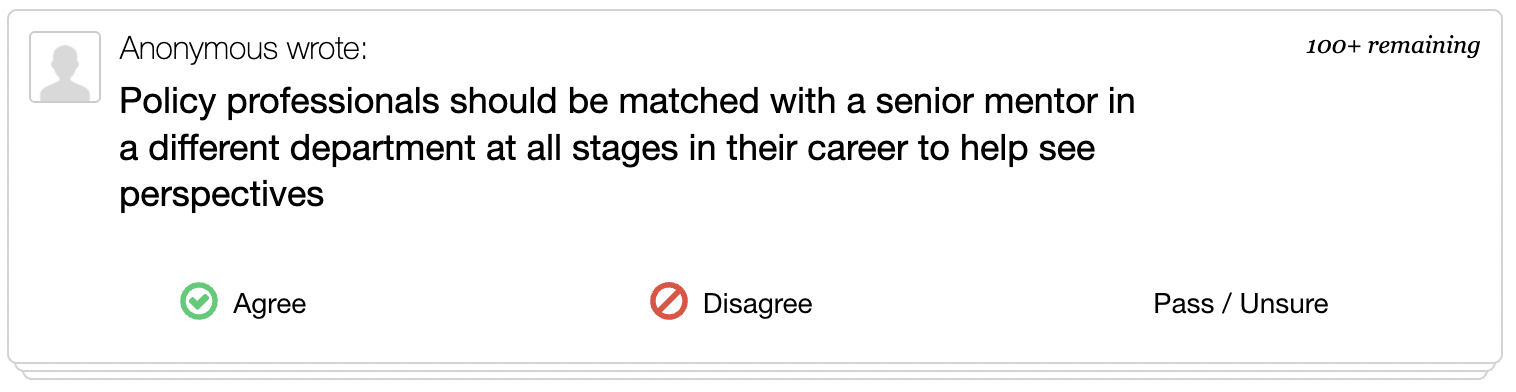
The software then uses machine learning to group participants according to how they vote on all statements in the debate.
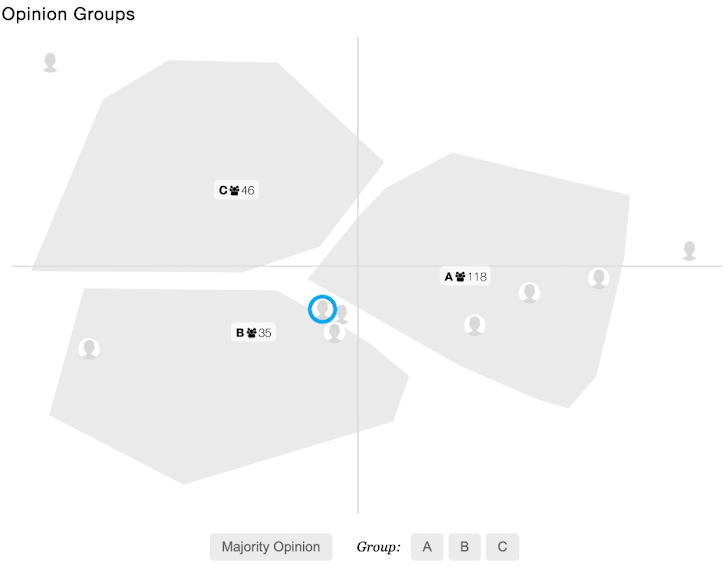
Building on our initial experiments in partnership with Demos, we have adapted our approach to using Pol.is in three ways by:
- convening a panel to moderate submitted statements to avoid duplicative or inappropriate content (this could include members of the policy team we are partnering with)
- collecting demographic data when participants register for a debate, if they consent. This allows us to further analyse the opinion groups generated by Pol.is to interrogate what people in a particular region, organisation or job role, for example, think about particular topics - whilst participants remain anonymous
- using Pol.is data to derive detailed personas for the opinion groups, or to draw policy implications that emerge from the discussion, depending on the policy team’s research focus
Collective intelligence for addressing complexity
From our experience using CILab on live policy issues, we have identified a series of policy use cases that echo findings from the government of Taiwan and organisations such as Nesta. These use cases include: 1) stress-testing existing policies and current thinking, 2) drawing out consensus and divergence on complex, contentious issues, and 3) identifying novel policy ideas.
1) Stress-testing existing policy and current thinking
CI could be used to gauge expert and public sentiment towards existing policy ideas by asking participants to discuss existing policies and current thinking on Pol.is. This is well suited to testing public and expert opinions on current policy proposals, especially where their success depends on securing buy-in and action from stakeholders. It can also help collate views and identify barriers to effective implementation of existing policy.
From the initial set of eight CILab policy debates, we have learnt that it is sometimes useful to design a ‘crossover point' into the process. This is where part way through a debate, statements submitted by policymakers, subject matter experts and members of the public can be shown to each other, in a bid to break down groupthink across those groups. We used this approach in a Pol.is debate on a topic relating to UK foreign policy, and think it could help test how existing policies on complex areas such as climate change or social care are perceived within and outside government.
2) Drawing out consensus and divergence on complex, contentious issues
Policy is often difficult to design when different groups of people - say across regions, sectors, or demographic characteristics - appear to disagree on the nature of a challenge, and/or on ways to address it.
Because tools such as Pol.is are well-suited to showing where groups of people agree, CI could be used to test where different policy options lie within the Overton Window - defined as the range of interventions that mainstream opinion considers politically acceptable. This could enable policymakers to:
- understand the extent to which people support different potential policy approaches. By inviting policymakers, experts and members of the public to debate a range of future policies on complex problems, we could better understand which interventions enjoy the most support, and which are least supported. As an example, independent newspaper Scoop.nz took a similar approach to exploring possible ways to reduce obesity in New Zealand. This would be especially useful when using Polis as part of a competitive or crowdsourcing approach to solving public problems, as described by Nesta and the United Nations Development Programme here
- discover how stakeholder groups such as local government, service providers and communities might respond to proposed new policies
3) Identifying novel policy ideas
In the face of emergent and complex challenges such as climate change, no individual or group can claim a monopoly on ideas for effective policy. By harnessing the ideas of a diverse group of people beyond those who traditionally contribute to policymaking, a CI exercise could help reveal new ideas to be tested. CI has already identified novel climate change solutions. For example, a teenage participant in the Climate CoLab, another CI initiative, won funding to scale up an innovative rainwater-powered sun-tracking solar panel through outreach in the developing world.
In our early experimentation in CILab, participants have proposed new policy ideas that had not previously emerged. Combined with traditional methods such as focus groups, and more innovative methods such as co-design, CILab debates can encourage participants to put forward new ideas for policy teams to develop into policies, services or other interventions.
Join in
If you think collective intelligence can help your team develop better policy in complex settings, please get in touch. We would love to work with teams across the public sector as we continue to learn how best to apply collective intelligence methods to policymaking.
2 comments
Comment by john mortimer posted on
'Policy is often difficult to design when different groups of people - say across regions, sectors, or demographic characteristics - appear to disagree on the nature of a challenge, and/or on ways to address it.'
Very true. If you ask service managers today, they will often express the issues that they face today and that will help alleviate the issues related to austerity. Not a good place to engage Policy.
To address linking policy to reality, the article does give some interesting ideas to clarify that link.
But there is another approach, and that is to get closer to the reality in the first place. Begin the process of Policy from that closeness. When we do that, the Policy then starts off from a much better place.
Comment by john mortimer posted on
I would say that developing innovative methods for Policy is primary around looking at the problem from different perspectives. What is the problem to solve and how do we solve it?
I say this, because there might be a thought that the gap between Policy makers, whose who do the service delivery, and the citizen can best be improved by more clever analysis. I am not sure.
What about bringing Policy people closer together with the problems that citizens have. What would be learn then?
What I have found is that if you ask citizens, they tell you what they have on their mind right now. And they tell you through their biases. If, on the other hand, you ask citizens at the point when they have the need for a service, then you will obtain the true nature of the demand at that time, and what matters to them then. That would be real information relevant to that service. repeat whatever time, and you have the knowledge you need.Over time that knowledge can then develop into trends and long term prevention.